By Chris Emezue
With the advent of AI and its ever-expanding applications, I have both witnessed and been at the forefront of fast-growing movements to harness artificial intelligence to address the unique challenges faced within the African context. This encompasses a broad spectrum of endeavors, ranging from efforts to enhance the representation of African languages in language technologies, education, training and awareness creation, to efforts to build tailor-made, AI-enhanced solutions that cater to the diverse needs of the African market. This embrace of AI cuts across multiple domains, with applications spanning sectors such as healthcare, finance, agriculture, linguistics or language, and law.
At the core of all these groundbreaking AI technologies, models, and systems lies a common bedrock: data (also called datasets), and therefore the success of these AI-based campaigns hinges on the collection, processing and modelling of data. Unsurprisingly, just like the recipe to a delicious meal, the data used, as well as the challenging process involved in its transformation, is the least discussed component when AI successes are advertised.
Africa faces many obstacles to adopting AI to solve its challenges, especially when implementation methods are simply copy-pasted from the Global North. One significant bottleneck to the effective implementation of “African-centric AI solutions” is the perceived lack of significantly large, high-quality, African-centric training datasets, brought about mostly by the lack of representation of African-centric content in the digital space. For example, ChatGPT, an AI chatbot language model developed by OpenAI, is said to have been trained ‘on the internet.’ However, African languages (2000+ of them) make up only about 0.1% of the languages represented on the internet (source). This means that the amazing capabilities of ChatGPT and other powerful models are not on par with African languages or generating African-centric content (the concept of a wedding ceremony in the US is different in Sudan, as demonstrated by Wafaa Mohammed in her Lanfrica Talk).
As we delve deeper into this problem, we uncover its multifaceted nature, revealing the complex mosaic of the data challenged on the African continent.
For one, there is the issue that while there have been (and still are) several efforts towards creating useful African datasets for AI and ML applications, many of these datasets are not very discoverable, living in dispersed repositories and servers and far away from the practitioners who can actually leverage them – a deep-rooted problem that Lanfrica Records, among other initiatives, is tackling. Moreover, there’s, understandably, a prevalent sense of wariness among data creators and holders when it comes to voluntarily sharing their data online. One such fear is exploitation. These challenges demand thoughtful and inclusive strategies to address data sharing challenged and foster trust, ultimately ensuring AI solutions are truly transformative for African communities.
Contrary to common perception, I believe the African continent is home to a treasure trove of invaluable data. What’s required is the establishment of mechanisms that facilitate the responsible sharing of this wealth of information. The key lies in enabling voluntary data sharing, both for the purposes of research and commerce. Analytics mechanisms also need to be in place to transform, process the data into useful information.
In our evolving digital landscape, the time has unquestionably arrived for a thoughtful discourse on the sharing of data. As the very nature of data continually transforms and diversifies, it becomes imperative to explore innovative approaches for enabling its voluntary sharing for research and commerce. This is eloquently elaborated upon in the Novmeber 2022 GPAI report, shedding light on the pressing need to adapt our strategies in the face of a changing data-driven landscape. As we embark on this journey, it is essential to recognize that the sharing of data is not just a technological matter but a crucial societal and economic consideration that has the potential to shape our collective future.
“Data farming” – a fundamental ethos when creating and sharing data.
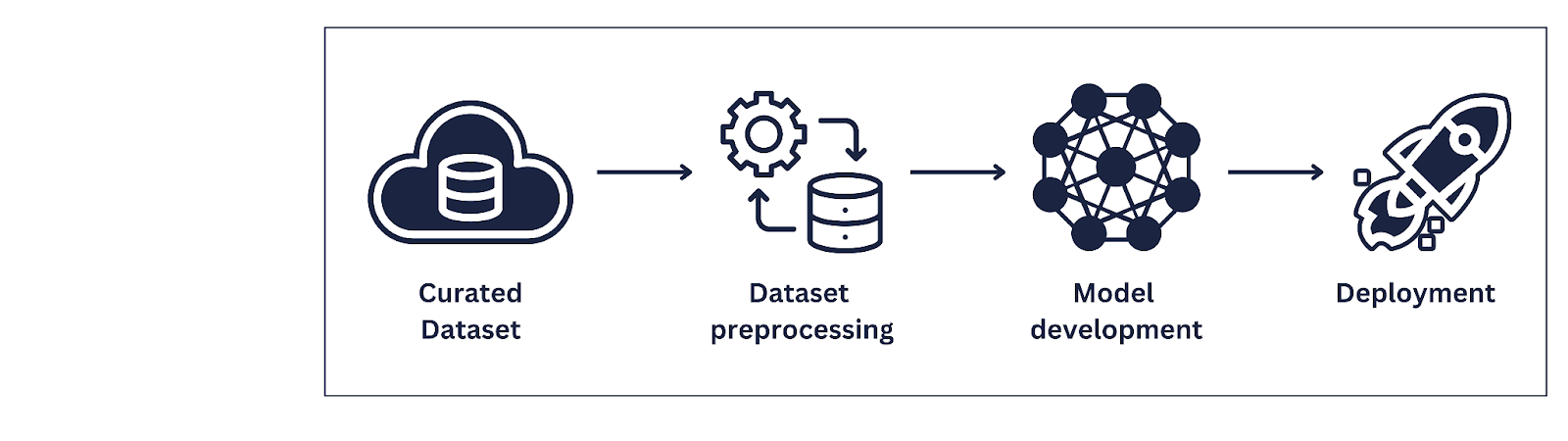
When we think of the machine learning pipeline, it typically starts with the data preprocessing or gathering and ends with the deployment of the application, with improvements done later on.
The concept of “data farming”, coined at Lanfrica, is an approach that represents a paradigm shift in the way data is gathered and managed. What distinguishes this approach is the inclusion of the data-providers, where the well-being and interests of the data-providing communities are thoughtfully integrated into the machine learning (ML) process.
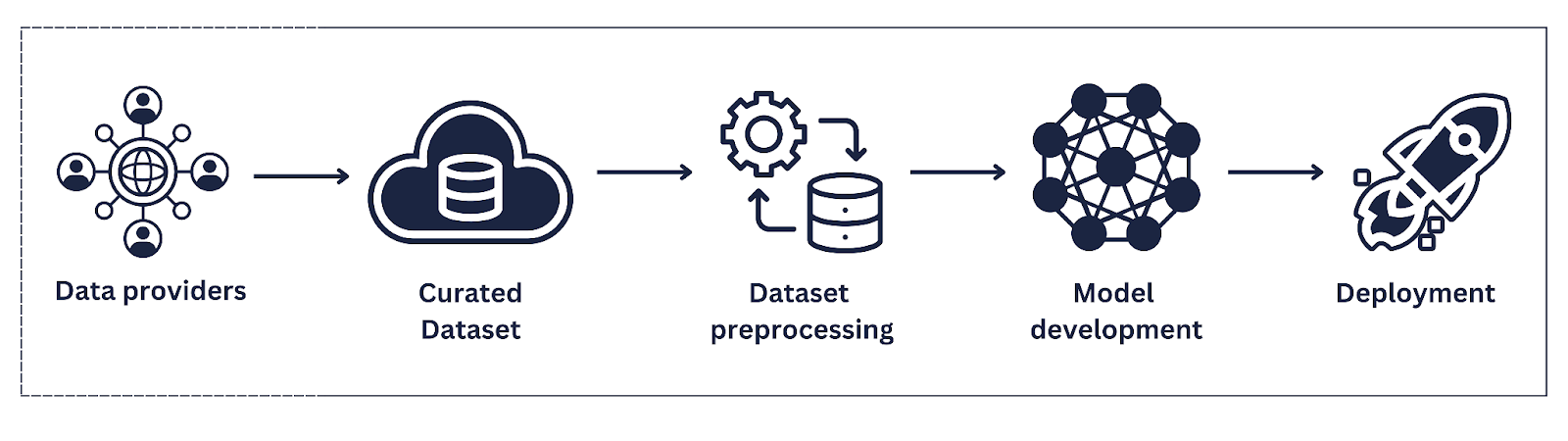
This principle ensures that 1) the data providers are included in the development of technologies, and 2) the benefits of data-driven advancements and AI technologies trinkle down to the data providing communities in one way or another. This emphasis on data farming, with its emphasis on community engagement and equitable practices, offers a promising solution to the challenges of data sharing, ultimately cultivating a more inclusive, responsible, and sustainable data ecosystem.
Some places where I have talked about data farming: